Article
What the ‘DeepSeek Moment’ Means for a CIO’s Long-Term AI Investments
5 essential, strategic moves for CIOs to navigate DeepSeek’s AI shift
February 20, 2025
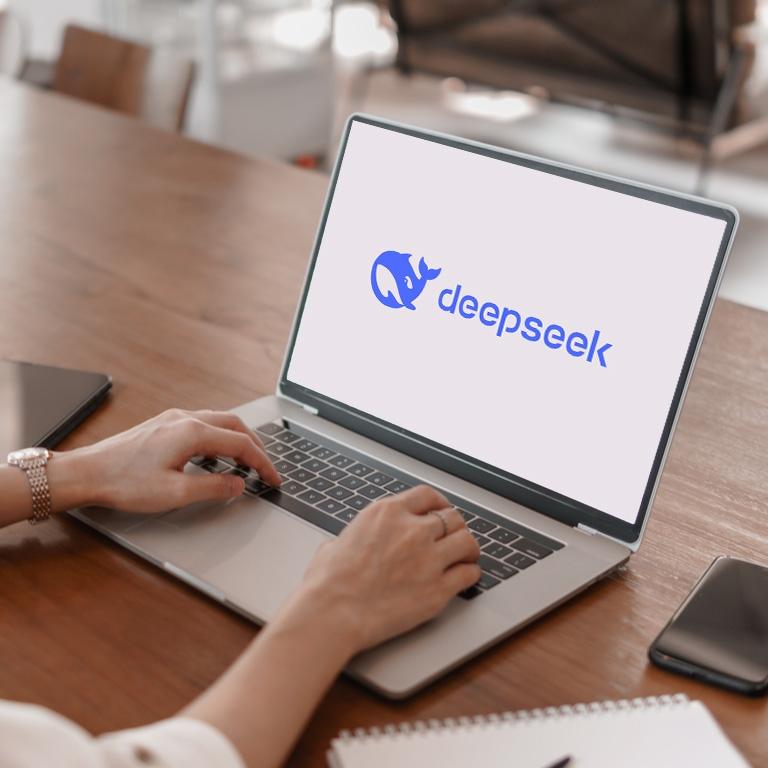
When DeepSeek introduced its model on Jan. 20, 2025, it significantly disrupted the AI hype cycle. It also introduced a new sense of urgency for CIOs around their AI strategies, deployment models, and cost structures. Why? It came in at a fraction of the cost while rivaling the tech capabilities of AI leaders like ChatGPT vs. Gemini.
Beyond the initial headlines, DeepSeek reflects something more significant. While it can be seen as “just another model launch,” it also signals the natural evolution of AI models toward commoditization – an evolutionary pattern we’ve seen before with something like cloud services.
As models become more efficient and cost-effective, organizations will have more AI options available. This means CIOs need to think strategically about how they select, implement, and manage these tools
Here are 5 key actions CIOs should take to adapt to the AI evolution driven by DeepSeek's arrival:
1. Design your technology architecture for AI model flexibility.
One key lesson organizations learned during the rise of cloud computing was the importance of flexibility in architecture. Those same principles now apply to AI implementation. Don’t bet everything on a single model or provider. Instead, build architectures that can adapt to changing needs and new capabilities.
In an ideal architecture, new models can be incorporated without needing to be completely rebuilt. This means organizations need to develop frameworks for testing and validating new models that can be applied consistently as new solutions emerge. And most importantly, organizations need to think about their AI capabilities as a spectrum of tools – not as a single solution.
The key is to maintain the ability to switch between models based on specific use cases while ensuring consistent security and performance standards. This approach allows organizations to take advantage of improvements in both cost and capability over time, without becoming locked into any single provider or solution.
2. Choose your AI deployment strategy based on value.
CIOs face a fundamental choice when it comes to AI deployment: is it better to go with a self-hosted open-source model, or to leverage managed services. Each approach carries different implications for security, cost, and control:
Self-Hosted Open Source | VS | Managed Services |
---|---|---|
Run models on your architecture | ⚡ | Faster implementation, but less control |
Full control over data and security | 🔒 | Data privacy concerns with data being routed externally |
Requires thorough code review and security vetting | 🛡️ | Lower operational overhead |
Higher operational overhead but better data sovereignty | ⚖️ | Higher ongoing costs, but faster time-to-value |
To most effectively make this choice, evaluate your AI initiatives against these key criteria:
- How sensitive is your data, and what are your regulatory requirements? Self-hosted solutions offer greater control but require more rigorous security implementation.
- How much customization will your use cases require? Self-hosted models allow deeper customization but demand more technical expertise.
- Does your team have the technical capabilities to manage open-source models? Self-hosted solutions require significant internal expertise for deployment and maintenance.
- What's your organization's appetite for operational and security risk? Self-hosted solutions give you more control over risk management but require robust internal controls and monitoring.
- How quickly do you need to implement AI capabilities? Managed services typically offer faster deployment but may limit long-term flexibility.
- What's your total cost threshold, including implementation, training, and maintenance? While managed services have higher ongoing costs, self-hosted solutions require more upfront investment in architecture and expertise.
3. Relentlessly focus on business value.
If you think the ROI of AI commoditization is just having access to cheaper models, you’re not thinking far enough ahead.
The real value of AI evolution is how business problems can be solved more effectively. As AI models become more accessible and less expensive, less time will be needed on how tools are implemented and instead focus on how these tools will most effectively be used.
Making this shift requires a practical approach to model selection and implementation. Successful organizations won’t be getting caught up in the technical specifications of new products. Instead, they’ll be focused on testing multiple models against specific use cases, grabbing low-hanging automation opportunities to free up resources for more complex problems, and – always key – maintaining the flexibility to switch models if and when a better option becomes available.
4. Build for Continuous Evolution
AI will continue to evolve, and organizations will need an architecture that can evolve with it.
This goes beyond technical capabilities and also includes ensuring processes and procedures can be adapted and improved over time. A new tool within an outdated architecture won't provide value.
Organizations will need efficient evaluation methods as new models emerge. This includes frameworks for assessing security implications and for monitoring and comparing performance. These frameworks need to be designed in tandem with clear criteria for how a model is eventually selected and implemented – including both technical capabilities and how they help to meet business needs.
The chief consideration for CIOs: The architecture they develop should be able to handle change without interrupting critical business operations.
5. Invest in Core Capabilities Within Your IT Team
Competitive advantage in the AI age will be about orchestration, not selection.
The organizations that invest in the right core capabilities – identifying valuable use cases, establishing strong security and governance frameworks, building robust integration capabilities, and developing internal expertise – will succeed in this ever-changing environment.
Conclusion
AI is not a collection of individual tools, but a dynamic capability. And while the emergence of a more efficient, cost-effective AI model like DeepSeek represents a significant development, it is not a reason to rush to adopt something new. The strategic imperative for CIOs isn’t to rush toward today’s most efficient model, but to build the right architecture and governance that can evaluate and implement new AI models and tools—quickly and strategically.
Authored By: Dhaval Moogimane, Sean McHale, and Erik Brown