Article
Bad data is costing your utility and undermining efficiency
Reliable insights start with clean data
October 31, 2024
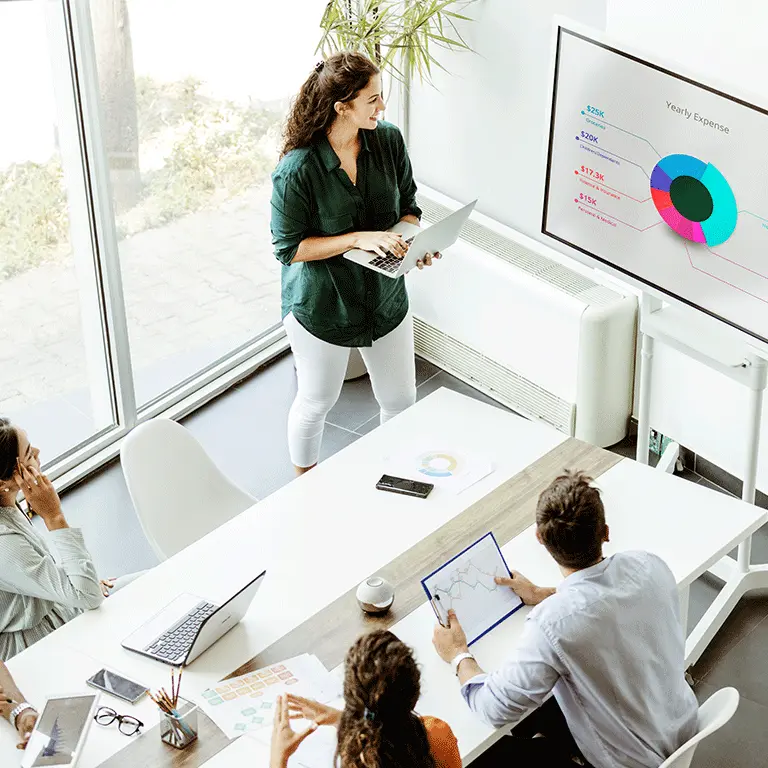
The accuracy and reliability of data can make or break an organization. Globally, data has grown year-over-year since 2010 and estimates project 181 zettabytes of data to be generated in 2025. And yet bad data is so pervasive that it costs U.S. companies a staggering $3.1 trillion annually.
Decisions based on flawed data can steer your business in the wrong direction. Inaccurate data skews analysis and insights, leading to strategies that fail to meet business goals. This can affect various aspects of the business, including marketing strategies and resource allocation.
Utilities collect diverse intake data from various sources, categorized into customer, operational, and environmental data, to optimize operations, enhance customer service, and ensure regulatory compliance.
The impact of poor data quality in utility operations cannot be overstated. It undermines efficiency, reliability, and cost-effectiveness, and utilities face significant challenges in delivering timely services and maintaining customer satisfaction without accurate intake data. Addressing these data quality issues is crucial before implementing any solutions to ensure seamless operations and optimal performance.
Before utilities set out to mine data, implement automation processes, integrate advanced data solutions, or engage in grid data sharing, they must first define their challenges—with a special focus upfront before data points are collected. And that’s something utilities must work through manually.
The impact of poor data quality
During COVID, disruptions in supply chains exposed inefficiencies and lack of resiliency in material and inventory management for our client, a large US electric and gas utility, costing them millions annually. They realized the need to identify pain points and explore solutions, including custom tools. Our research revealed that poor data quality stemmed from multiple systems and tools that were inconsistently used. Leaders didn't trust the data, often relying on estimates from previous years, resulting in a lack of confidence in action plans.
The Response
After collaborating with our design and engineering teams, we collaboratively developed a custom tool to address data breakdowns. This tool provides a comprehensive view of material demands across all levels, improving their ability to plan and respond to events without inflating inventory. Leaders now trust the data, enabling them to build forecasting models for 2-5 years ahead, something they couldn't do before. This newfound data reliability is saving millions, boosting employee morale, and empowering forecasting and planner roles.
“Embracing the power of good data has transformed our approach entirely. What was once a conversation solely about data quality has evolved into harnessing its full potential to drive unparalleled value. Our newfound focus on demand verification and validation has sharpened our decision-making, ensuring every action is rooted in accurate insights.” - Stakeholder
Advancing utility collaboration through the power of AI
Continuing to collaborate with utilities, the focus is on better understanding where they can lean in, especially with advancements in Generative AI. This technology rapidly processes data from various sources like facility operations, weather patterns, geographic information systems, and cybersecurity threats. As AI models continue to evolve, they require robust human oversight to ensure responsible use.
Pilot programs already demonstrate Generative AI's ability to design customer engagement campaigns for utilities. These initiatives showcase its potential for enhanced interaction with infrastructure—from substations and control rooms to transmission lines and distributed energy resources like microgrids.
Based on work with utilities throughout their data lifecycle, here are some essential questions every utility should be asking before they start their data journey:
- Why are you collecting data?
- What data do you need?
- How will the data look?
- How do you get the data?
Strategic questions for high-quality intake data
Ensuring quality intake data is crucial for any organization aiming to leverage advanced technology solutions for strategic decision-making and operational efficiency. This is particularly important in the utility industry, where the significance of data quality is rapidly growing with new analytics use cases.
The potential expansion of grid data sharing on a national level further elevates the magnitude for sound data. Advances in data science and artificial intelligence are creating opportunities for business improvement and innovation, and integral processes such as asset management, grid optimization, cybersecurity, environmental management, demand forecasting, regulatory compliance, decarbonization, renewable energy integration, and energy storage management can all benefit.
Defining quality is essential, whether data is collected manually by field personnel or through automated devices. Recognizing the complexity and importance of this definition is the first step for utilities. Establishing stage gate criteria and ensuring accountability should follow.
Data capture detail varies by use case but can be determined by addressing the purpose and setting the framework for optimal capture. For example, conducting detailed field assessments of grid infrastructure after a natural disaster to initiate repairs requires assessors to collect material-level condition data to accurately inform engineering—detailing obvious structural damage may suffice for prioritizing emergency construction activity immediately after a disaster. In both scenarios, the right questions must be asked to inform decision-making, collection strategy must be tailored, and the data capture mechanism must be configured to gather the necessary information. Utilities can achieve this through dedicated cross-disciplinary collaboration, securing a clear understanding of data use cases.
When the definition of “quality” is organizationally clear you can implement a quality assurance process, encompassing data governance, standards, training, and validation. The following inquiries help develop strategies that drive integrity, accuracy, and reliability of data collected upfront.
A crucial aspect of answering these questions lies in training and adoption. Ensuring that all stakeholders are well-trained on the data collection tools and processes is essential for maintaining data quality and consistency. Training programs should cover best practices for data entry, the use of validation tools, and the importance of adhering to data standards. This not only equips employees with the necessary skills but also fosters a culture of data quality across the organization. This approach not only enhances the user experience for field staff but also ensures that data entered into downstream systems—such as asset management—is reliable and actionable.
Adoption is equally important. Even the best tools and strategies will fall short if they’re not embraced by those responsible for data collection. Encouraging adoption through continuous support, feedback mechanisms, and incentives can significantly enhance the effectiveness of data collection efforts. By focusing on training and adoption, you drive efficient data collection processes that are also sustainable in the long term.
Conclusion
Ensuring quality data upfront is not just a best practice; it’s an imperative for any organization aiming to leverage advanced analytics, efficient data processing, accurate reporting, and robust storage solutions.
By addressing data quality issues at the source, utilities can prevent the cascading effects of inaccuracies that compromise decision-making and operational efficiency. Utilities aiming to assess and implement custom-built or tailored digital experiences for field personnel need a culture of confident data intake, serving as the foundation for seamless integration into the system of record, facilitating accurate and efficient end-to-end data collection.
There is no mystery in reducing the costs of bad data — it requires a proactive approach to defining and collecting data meticulously before it enters the data lifecycle. This upfront investment in data quality not only mitigates downstream challenges but also unlocks the full potential of data-driven initiatives, paving the way for more reliable insights, enhanced performance, and sustained value creation.