Article
The Growing Role of Humans in the Loop for Smarter Data Governance
As AI expands data oversight, human expertise remains essential for trustworthy governance
January 24, 2025
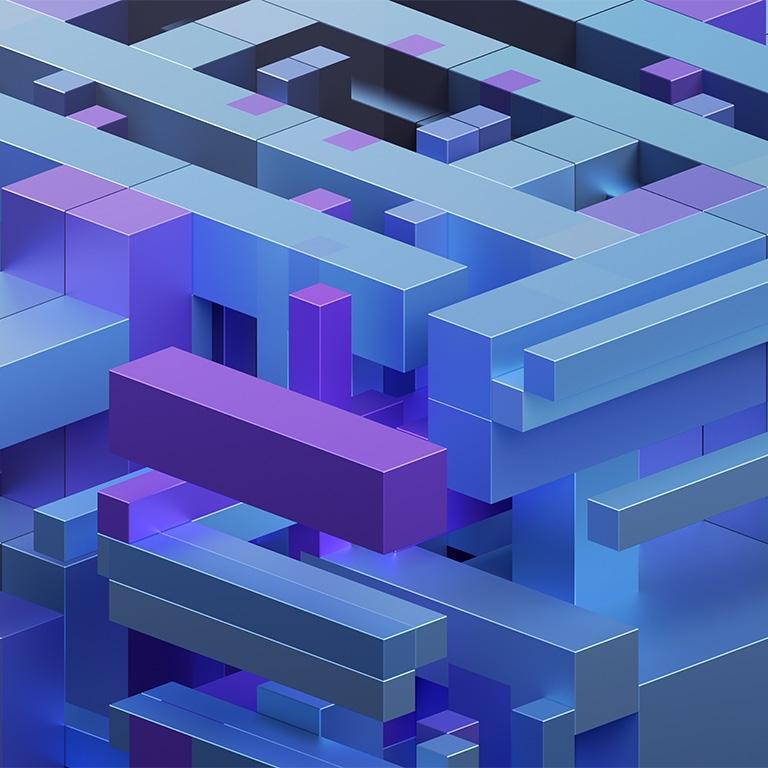
It’s no secret that the rise of generative AI (GenAI) has touched every corner of the data and analytics landscape. We consistently hear a common refrain: “We need good data to enable AI.” This puts considerable pressure on data engineering teams—especially in an era of tightened budgets—to innovate and support AI initiatives. It also places renewed emphasis on ensuring organizational data is high-quality, accurate, readily available, and well-understood.
This sharpened focus on quality has made data governance far more than a nice-to-have. While data governance has long been critical for ensuring data consistency, quality, and regulatory compliance, its importance is magnified as organizations adopt more AI-driven technologies.
Conversely, AI itself is reshaping how data governance processes work, introducing new efficiencies and capabilities. Tools dedicated to master data management, data cataloging/metadata management, data quality, and governance workflows are getting significant upgrades—helping teams streamline administrative tasks and do more with less.
While the world is enthralled by AI’s capabilities, it’s impossible to overlook the “human in the loop” aspect within data governance; that human component is essential to a program’s success. Such transformation has substantial implications for data governance teams, particularly concerning the roles of data governance managers and data stewards.
These roles aren’t being replaced; they’re becoming even more indispensable in light of both the expanding significance of data itself and the higher stakes tied to AI-driven decisions.
Data governance: The backbone of AI initiatives
Data governance frameworks and structures form the bedrock for AI systems to effectively operate. If data is incomplete, biased, or inaccurate due to weak governance, AI outcomes can easily veer off course, leading to flawed outcomes and decision-making. As organizations apply AI across essential business functions, it’s never been more important to ensure data reliability through governance.
In practice, data governance frameworks ensure that data policies are in place, roles and responsibilities are clearly defined, and data quality, standards, and security are consistently upheld. By instituting these guardrails, organizations can trust that their AI models are ingesting accurate, trustworthy data—yielding insightful, actionable intelligence with reduced risk of error or bias.
AI-driven data governance: Enhancing processes, at scale
On the other side of the equation, AI is being threaded into many data governance activities, automating and optimizing tasks such as data quality checks, record survivorship, data classification, data lineage tracking, and policy enforcement. The very characteristics that make AI so powerful in business scenarios—its capacity to sift through massive datasets, detect patterns, and spot anomalies—enhance an organization’s ability to govern data at scale. This is especially relevant for increasingly complex, hybrid, and multi-cloud environments where traditional manual oversight can no longer keep pace.
The AI impact on key data governance technology
According to recent market evaluations, seven out of Gartner’s top 10 MDM solutions incorporate AI for data matching, deduplication, enrichment, classification and validation, data quality checks, and even uncovering hidden relationships. By doing so, they reduce the manual workload required of governance teams. But as data governance tools (i.e., Master Data Management tools, Data Quality tools, Data Catalogs, Data Governance Workflow tools) become more sophisticated, human oversight remains vital. Data stewards must validate automated outcomes to ensure the organization’s data (master data, reference data, metadata, etc.) stays both accurate and reliable.
Master data management (MDM) solutions, designed to maintain a single source of truth for crucial data, are rapidly integrating AI features to boost overall efficiency. AI-driven MDM automates intricate processes like entity resolution, data deduplication and survivorship, and data enrichment, enabling faster and more consistent results.
Data catalog and quality tools, which are fundamental to organizing, classifying/profiling, and managing enterprise data—are incorporating AI to streamline operations further. AI-driven data cataloging automates the discovery of new data sources, applies smart tags, and tracks data lineage in real time, making it far easier for organizations to handle the complexity and volume of modern data landscapes.
West Monroe Spotlight: Data Quality Accelerator utilizes Gen AI for Deduplication
DQA (Data Quality Assessment) is an accelerator built by West Monroe, leveraging Databricks, that includes a comprehensive suite of data profiling tools as well as Gen AI-powered record-level data.
Gartner’s research indicates that eight of the top 10 data catalog solutions leverage AI to facilitate data classification, lineage discovery, automated tagging, relationship mapping, data quality monitoring, search functionality, data dictionary creation, and policy enforcement. This broad adoption demonstrates how AI helps reduce the day-to-day burden on data governance teams.
Still, human stewards (both business and technical) play a critical role in overseeing AI-driven tools. They’re responsible for defining the business rules that drive these tools, validating the accuracy of outputs (survived records, data classifications, etc.), and preventing potential governance failures that could emerge if AI-driven policies go unchecked.
West Monroe Spotlight: AI Data Steward
While various data catalogs are implementing Gen AI features to help enrich metadata, this is not yet fully available within all catalogs. This still leaves data stewards with heaps of manual work that often slows down data catalog adoption.
By leveraging West Monroe’s own Gen AI Platform ‘Nigel’, we created the data governance accelerator ‘AI Data Steward’, which can generate data descriptions and glossaries for data assets that can be imported within all data catalogs, greatly accelerating normally resource-intensive data stewardship activities.
While AI lightens the manual workload for governance teams, it can also introduce new risks. Automated decisions on policy enforcement—if not properly supervised—can accumulate errors that may have wide-ranging effects. For example, a misclassified dataset might slip through compliance safeguards, triggering audits or penalties and undermining trust in your data ecosystem.
This is precisely why the need for human data stewards hasn’t diminished; it has become more urgent and pronounced.
The Role of Data Stewards in an AI-driven governance world
As AI takes on a broader role in data governance, the demand for highly capable data stewards also rises. Data stewards are tasked with ensuring data quality, overseeing adherence to policies, and making critical decisions on data usage. While AI can assist by automating many mechanical processes, it cannot replicate human judgment, domain context, or ethical oversight—elements that are fundamental to robust data governance.
Where AI-driven governance falls short is often in recognizing context or moral nuances. A single miscalculation can affect massive volumes of data, leading to compliance infractions, operational setbacks, or even reputational damage. Data stewards offer a safety net, continuously monitoring AI-driven operations to confirm that decisions align with the organization’s standards and regulatory obligations.
Ultimately, the human oversight provided by data stewards balances AI’s scale and speed with crucial ethical and business contextual considerations—essentials for safeguarding quality and compliance in data governance.
The impact on data governance teams and the rising importance of stewardship
In the age of AI, skilled data stewards are more critical than ever. While AI can handle a significant portion of the grunt work, data stewards retain the responsibility of applying human insight, ethical considerations, and specialized domain knowledge to confirm that AI-driven governance delivers correct, compliant, and beneficial outcomes. Errors at scale can carry serious consequences, which makes a watchful, human-centric approach essential for mitigating risks and aligning with broader organizational goals.
The merging of AI into data governance frameworks unlocks immense potential—streamlined processes, faster insights, and broader scalability. But these benefits come with heightened stakes. Governance teams must adapt quickly, emphasizing stewardship and informed decision-making even as AI automates much of the routine. In an AI-powered world, the role of data stewards has never been more central to safeguarding the integrity of data governance and ensuring overall success.
By: Nick Burchard and Annemieke De Groot